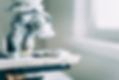
USING MULTIPLE MACHINE LEARNING CLASSIFIERS TO EXPLORE HUNTINGTON'S DISEASE AND MICRORNAS

Abstract
Huntington’s disease is a progressive neurodegenerative disease caused by a mutation in the HTT gene. Although there are treatments targeted towards handling the symptoms, none can cure the disease or even slow down its progression. MicroRNAs (miRNA) are small non-coding RNA molecules that regulate gene expression. Since the suppression of mutant genes can cure or slow down the progression of a disease, microRNAs can potentially be used as a treatment option for Huntington’s disease. However, in order to do so, a relationship must be established. Machine learning algorithms have the potential to uncover patterns that are hard to pick up by humans. Therefore, this project aimed to use different machine learning approaches to examine the relationship between Huntington’s disease and microRNA expression data in blood. It was hypothesized that if deep neural networks, random forest, gradient boosted decision trees, and support vector machine algorithms were implemented, then all of the models would perform better than the dummy classifier (representing random guessing), with the deep neural network achieving the highest accuracy. Each classifier was trained and the average of the area under the receiving operating characteristic curves of 100 iterations was plotted. As hypothesized, all of the classifiers performed significantly better than the dummy classifier with a p-value less than 0.05 for each. However, the support vector machine did unexpectedly better than the other models. The results indicate the existence of a relationship between miRNAs and Huntington’s disease, which can potentially be used after further research to synthesize a treatment.
Project By: Deeksha Kumaresh
Current sophomore at American Heritage Boca/Delray
Using different machine learning classifiers to examine the relationship between prodromal Huntington’s disease and miRNA expression in blood